Organizers
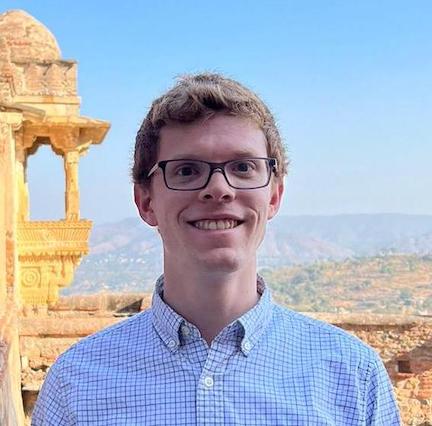
Adam Block is a postdoc at Microsoft Research NYC. In July 2025, he will join the Department of Computer Science at Columbia University. Previously, he was a PhD student in the math department at MIT with affiliations in the Laboratory for Information & Decisions Systems and the Statistics and Data Science Center. His research focuses on bridging theory and practice within machine learning by designing efficient algorithms with provable guarantees under realistic assumptions on data, with a special focus on learning in sequential decision making tasks. His research was generously supported by an NSF Graduate Research Fellowship. Before MIT, Adam completed a B.A in Mathematics at Columbia University, where he was an I.I. Rabi scholar.
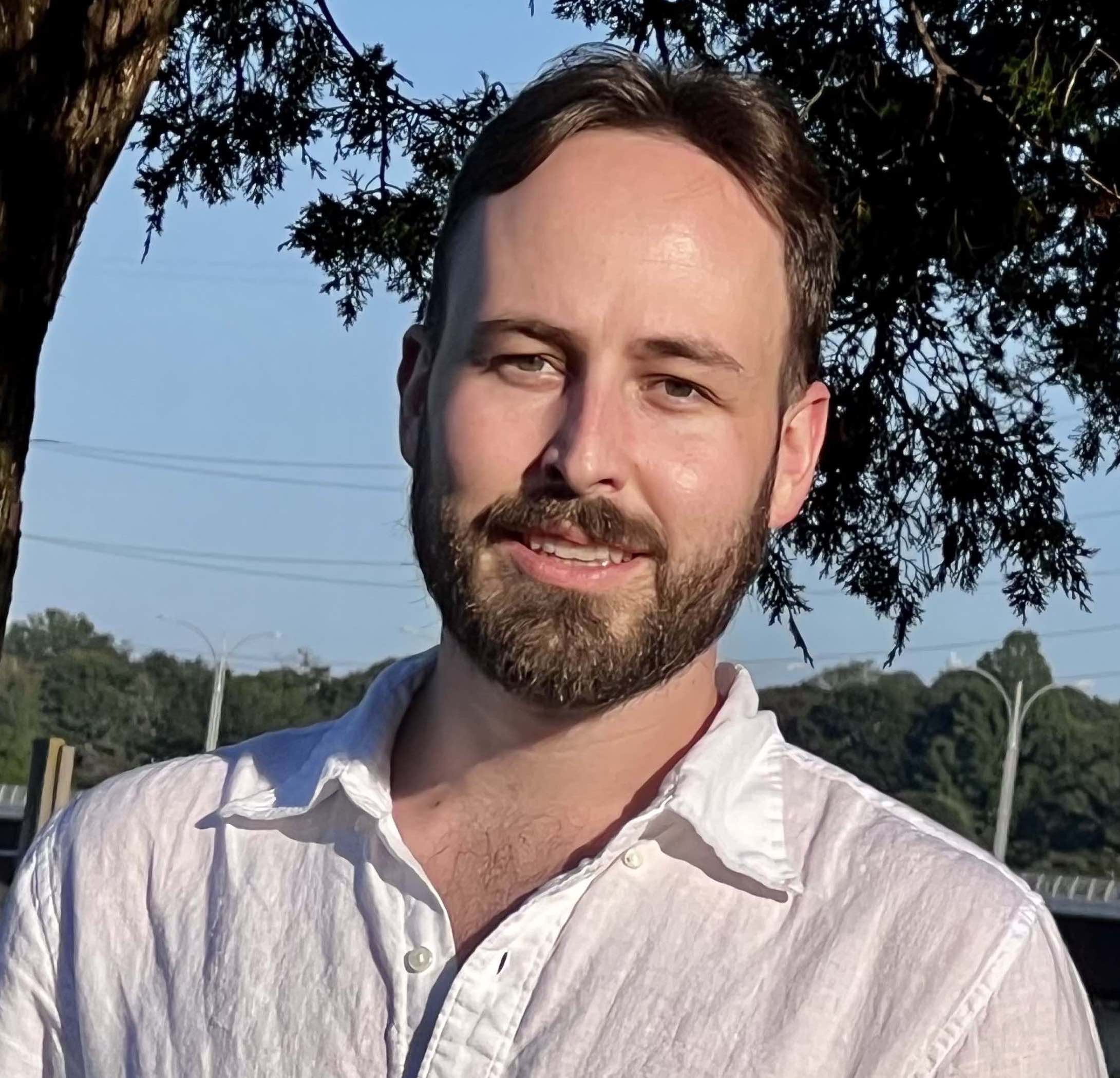
Dylan Foster is a principal researcher at Microsoft Research, New England (and New York City) where he is a member of the Reinforcement Learning Group. Previously, he was a postdoctoral fellow at the MIT Institute for Foundations of Data Science, and received his PhD in computer science from Cornell University, advised by Karthik Sridharan. His research focuses on problems at the intersection of machine learning, AI and interactive decision making. He has received several awards for his work, including the best paper award at COLT (2019) and best student paper award at COLT (2018, 2019).
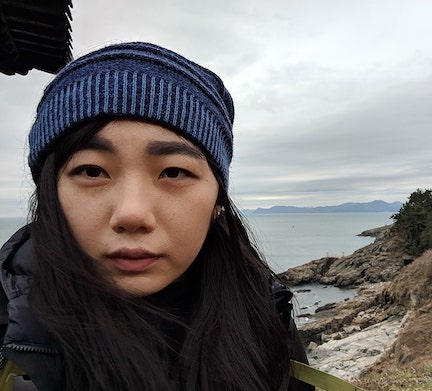
Audrey Huang is a fourth-year PhD candidate in Computer Science advised by Nan Jiang at the University of Illinois Urbana-Champaign. Currently, she is excited about combining ideas from RL theory with the capabilities and structures of language modeling to design efficient and provable algorithms. She also works on the complexity of online/offline RL with function approximation, and imitation learning. Audrey has interned at Microsoft Research, Google, and Adobe, and received her MS from Carnegie Mellon University and BS from Caltech.
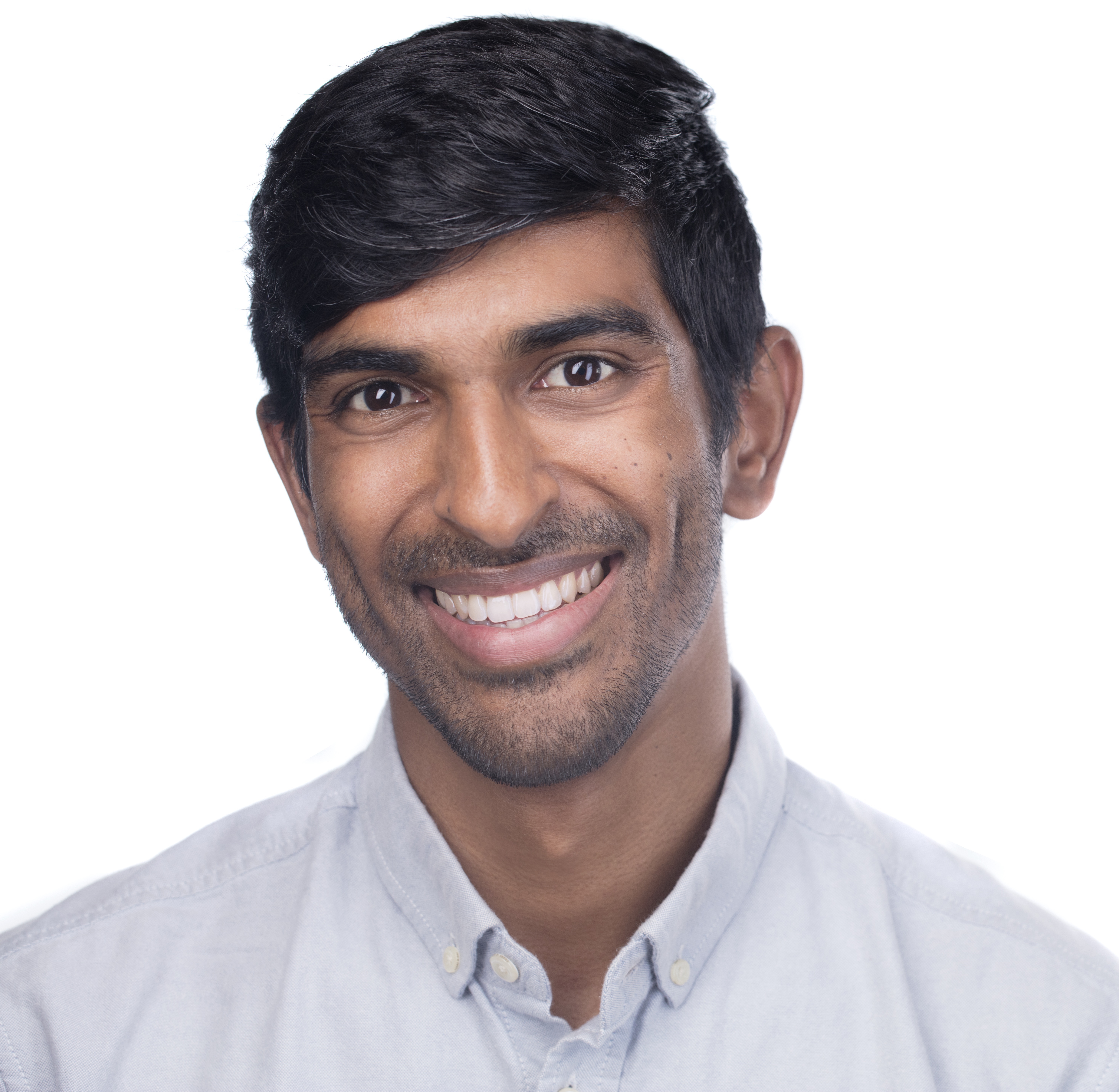
Akshay Krishnamurthy is a senior principal research manager at Microsoft Research, New York City. Previously, he spent two years as an assistant professor in the College of Information and Computer Sciences at the University of Massachusetts, Amherst and a year as a postdoctoral researcher at Microsoft Research, NYC. His research interests are in machine learning and statistics, with a focus on interactive learning, or learning settings that involve feedback-driven data collection. His recent interests revolve around decision making problems with limited feedback, including contextual bandits and reinforcement learning.
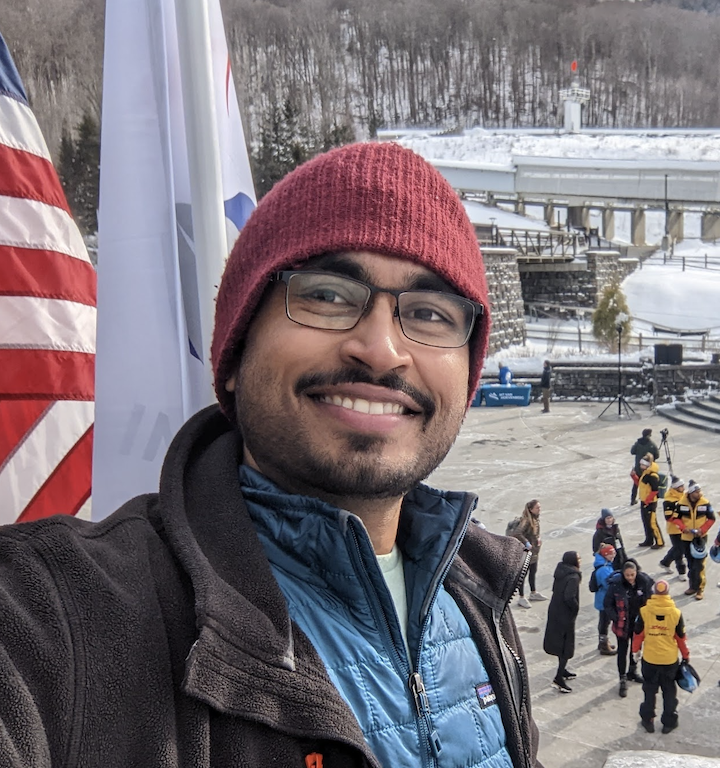
Nived Rajaraman is a final year PhD student at UC Berkeley, jointly advised by Jiantao Jiao and Kannan Ramchandran, affiliated with the BLISS and BAIR labs. His research focuses on a variety of topics in the theory of machine learning with a general focus on the statistical and computational aspects of adaptive decision making and reinforcement learning, recently extending to applications advancing our understanding of large language models. He has contributed to the academic community as an organizer of the BLISS and CLIMB seminars at Berkeley.
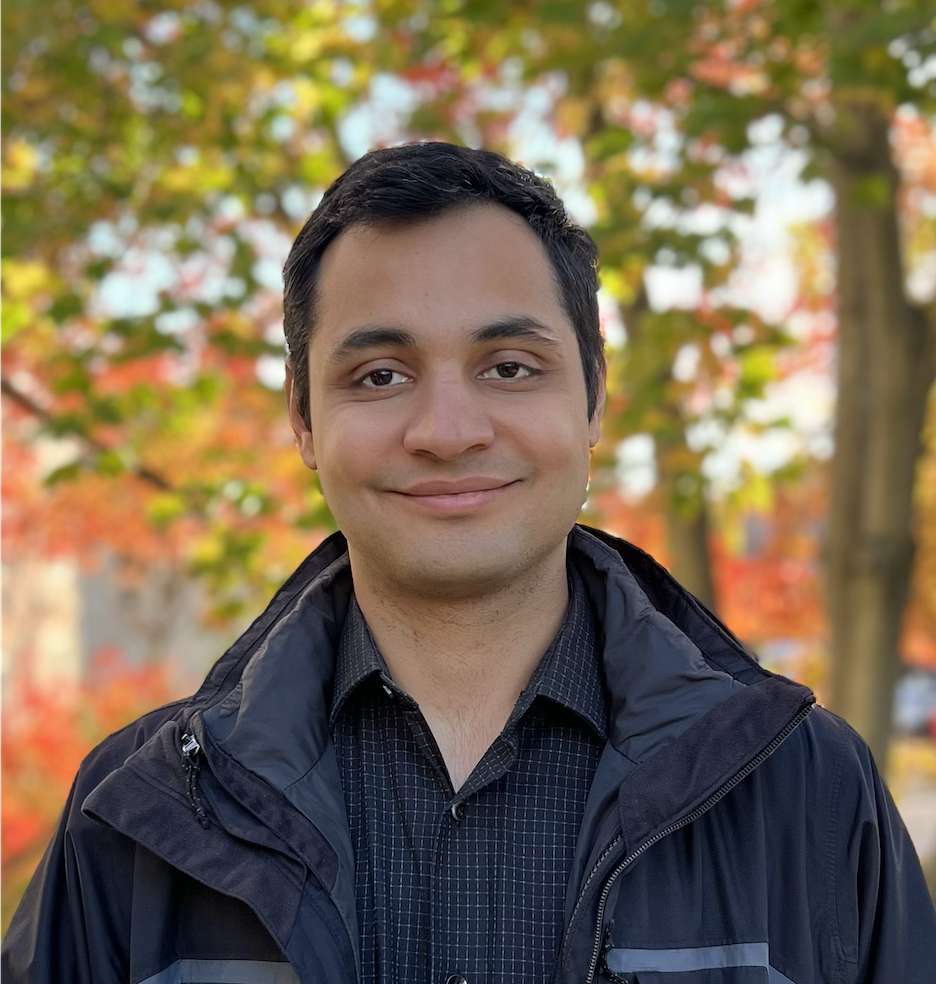
Ayush Sekhari is a postdoctoral researcher at MIT working with Prof. Alexander Rakhlin. Ayush received his Ph.D. from Cornell University, advised by Professor Karthik Sridharan and Professor Robert D. Kleinberg. His research interests span optimization, online learning, reinforcement learning and control, and the interplay between them. Before coming to Cornell, he spent a year at Google as a part of the Brain residency program. Before Google, he completed his undergraduate studies in computer science at IIT Kanpur in India where he was awarded the President’s gold medal. His work has been recognized by a best student paper award at COLT (2019). He also serves the community as an organizing committee member of the Learning Theory Alliance.